From nanoelectrode arrays that peer inside heart cells to single-layer spintronics that manipulate quantum states, researchers are advancing how we observe and control matter at multiple scales. This roundup examines breakthroughs in non-invasive cardiac monitoring and AI-powered genomic modeling, alongside developments in energy-efficient computing through anomalous Hall torques. Also in this week in AI and semiconductors, Mayo Clinic’s partnerships with Microsoft Research and Cerebras Systems aim to build foundation models for medical imaging and genomic analysis, while Penn Medicine’s MISO platform processes up to 30,000 data points per pixel for enhanced cancer detection.
AI in healthcare
AI-driven method offers noninvasive peek inside heart cells
Researchers at the UC San Diego and Stanford have developed a technique that reconstructs the electrical activity inside heart cells without the need for invasive probes. In the research, described in a study in Nature Communications, stem-cell-derived heart cells were grown on nanoelectrode arrays. Next, the scientists relied on thousands of paired intracellular and extracellular signals to allow a deep learning model to infer subcellular events from surface data alone. “We discovered that extracellular signals hold the information we need to unlock the intracellular features that we’re interested in,” said Zeinab Jahed, a UC San Diego professor, in a press release.
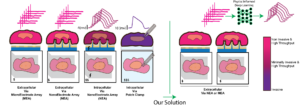
Comparing methods for recording signals within cells. Existing approaches (left) are expensive, highly invasive, and limited in throughput. A new technique (right) uses AI to reconstruct intracellular signals from less invasive extracellular recordings. [Images by Keivan Rahmani.]
At the heart of the approach are “nanoelectrode arrays, which can simultaneously record intracellular and extracellular signals from thousands of interconnected cells” and PIA-UNET, which the paper notes “enables fast and precise reconstruction of iAP signals.” Physics-Informed Attention-UNET (PIA-UNET) is a deep learning model that combines traditional neural network architecture with physics-based constraints to accurately reconstruct intracellular action potential waveforms from extracellular recordings. It incorporates both data-driven learning and electrophysiological principles.
This approach could reduce the reliance on invasive methods for testing new heart drugs, offering a more efficient route to assess drug toxicity. The researchers demonstrated the technique’s potential for high-throughput drug screening using stem cell-derived cardiomyocytes and aim to expand this technology for use with neurons and other cell types.
Mayo Clinic, Microsoft Research and Cerebras to partner on foundation models for personalized medicine
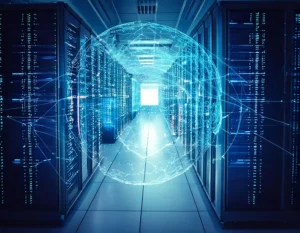
[Adobe]
Mayo Clinic recently announced two major collaborations with Microsoft Research and Cerebras Systems to build “foundation models” for medical imaging and genomic data analysis. These large-scale AI models would be able to swiftly adapt to specific clinical tasks, potentially boosting radiology capabilities through automated report generation and more precise image interpretation to improve clinician workflow and patient care. The multimodal foundation can tackle “significant roadblocks across the radiology ecosystem.” noted Dr. Matthew Callstrom, chair of Mayo Clinic Radiology in the Midwest and medical director for Generative AI and Strategy, in the press release.
Meanwhile, Mayo Clinic’s partnership with Cerebras focuses on creating a genomic foundation model using both patient exome data and public genome references. Early studies indicate this approach may reveal disease patterns and therapy responses more quickly than standard methods, proving especially valuable for complex conditions like rheumatoid arthritis. The alliance was unveiled at the J.P. Morgan Healthcare Conference.
AI tool ‘MISO’ mines thousands of data points per pixel to improve cancer detection
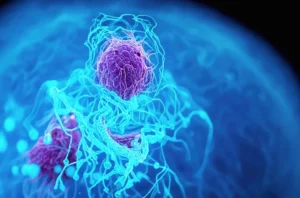
[From https://www.pennmedicine.org/]
At the Perelman School of Medicine at the University of Pennsylvania, researchers have introduced MISO (Multi-modal Spatial Omics), an AI-based platform that processes up to 30,000 data points within a single tiny pixel of cancer tissue. As the researchers note in their Nature Methods paper, “Spatial molecular profiling has provided biomedical researchers valuable opportunities to better understand the relationship between cellular localization and tissue function.” This insight is important l because, as the researchers emphasize, “effectively modeling multimodal spatial omics data is crucial for understanding tissue complexity and underlying biology.” MISO offers more detailed insights than conventional scans, helping clinicians pinpoint crucial information about how varied cell populations within tumors respond to therapies. Already, the platform has flagged specific cell clusters correlated with better immunotherapy outcomes in bladder, gastric, and colorectal cancers.
By integrating spatial omics data—such as transcriptomics and proteomics—MISO constructs a map that links the physical layout of tumor tissue with its molecular makeup. Future plans involve scaling the platform to handle larger datasets and additional data types, including epigenetic markers.
AI model from Case Western Reserve aims to predict heart failure risk from CT scans
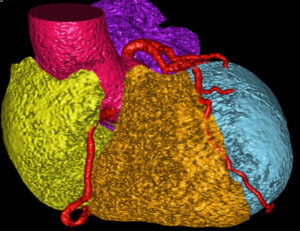
Using AI, physicians could better predict cardiovascular risk by analyzing CT scans and integrating patient biometric data. This image, courtesy of a Case Western Reserve University press release, illustrates how AI distinguishes different cardiac segments, such as the left and right atria, left and right ventricles, and cardiac arteries.
Investigators at Case Western Reserve University, University Hospitals, and Houston Methodist received a $4 million National Institutes of Health grant to create an AI tool for predicting cardiovascular events. The tool will use routine CT scans, specifically calcium-scoring CT, to analyze existing scan data and patient metrics. This analysis aims to identify risk factors by revealing coronary artery plaque accumulation, providing crucial insights into cardiovascular health.
By integrating large-scale imaging datasets with comprehensive clinical risk factors (including age, body composition, and demographic data), the research team aims to enhance physicians’ ability to identify and manage patients at elevated risk for cardiovascular disease. The technology makes use of existing screening protocols from both Houston Methodist and University Hospitals. It offers a non-invasive approach that could enable healthcare providers to implement more targeted preventative treatments and optimize patient outcomes.
The research team will develop AI-driven predictive models that analyze coronary calcium levels, heart shape, bone density, and visceral fat measurements to provide comprehensive cardiovascular risk assessments.
ALAFIA introduces on-prem HPC to power clinical AI workflows

[ALAFIA]
We caught up with ALAFIA Supercomputers at the JP Morgan Healthcare Conference to learn how the company is working to meet the high computational demands of AI applied to radiology, pathology, and advanced research by offering “personal supercomputers.” The company’s AIVAS system, for example, bundles up to 2 TB of ECC memory and tens of thousands of GPU cores, allowing local processing of large imaging datasets.
This HPC-based approach arose from hospitals’ reliance on older computing infrastructure to drive modern imaging equipment costing millions of dollars. Cloud-based GPU rentals, while flexible, can quickly generate high monthly fees when labs process thousands of slides daily. By keeping data on-prem and tapping significant on-board compute resources, ALAFIA systems enable local analytics with reduced latency and lower recurring costs. Hospitals also benefit from a long-term software maintenance guarantee (12 years through Canonical), helping ensure that intensive AI workflows—ranging from cell segmentation to volumetric imaging—remain feasible on-site for both clinical care and research.
Emerging tech spotlight
Researchers unveil ‘brand new physics’ for next-generation spintronics
Physicists from the University of Utah and the University of California, Irvine, have reported a new spin–orbit torque phenomenon known as the anomalous Hall torque, which may pave the way for ultra-fast, energy-efficient computing devices. Traditional spintronic approaches typically rely on multiple magnetic layers to flip electron spins, but this discovery demonstrates how a single layer of ferromagnetic material can transfer spin currents, potentially removing the need for multi-layered structures. By tuning symmetry and leveraging Hall-like effects, the team can precisely control electron spins, offering a promising path for high-speed data storage and neuromorphic computing. “This is brand new physics, which on its own is interesting, but there’s also a lot of potential new applications that go along with it,” said Eric Montoya, assistant professor of physics and astronomy at the University of Utah, in a press release. “These self-generated spin-torques are uniquely qualified for new types of computing like neuromorphic computing, an emerging system that mimics human brain networks.” The research was published in Nature Nanotechnology.
The study adds to the “Hall triad” of spin-orbit torques—encompassing the spin Hall, planar Hall, and now anomalous Hall torques—which appears to be broadly applicable across all conductive spintronic materials. Having already constructed a prototype spin-torque oscillator to demonstrate the effect, the researchers plan to link multiple nanoscale oscillators into larger networks for tasks such as image recognition. This approach could lead to a new generation of computing devices that operate at higher speeds and with fewer energy demands than current hardware.
Tuning Magnetism with Voltage Opens a New Path to Neuromorphic Circuits
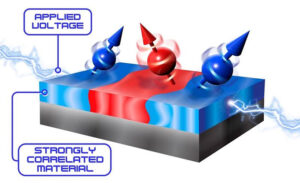
Applying a voltage to lanthanum strontium manganite (LSMO) causes it to separate into regions with dramatically different magnetic properties, represented by the red and blue areas in the figure. [New York University and the University of California San Diego/US DOE]
A quantum material called LSMO can be split into magnetic “regions” using an applied voltage, paving the way for energy-efficient spintronic neuromorphic devices.Researchers at the U.S. Department of Energy have demonstrated that applying a small voltage to lanthanum strontium manganite (LSMO)—a quantum material known for its transition between metallic/magnetic and insulating/nonmagnetic states—induces the formation of distinct magnetic phases. Each phase resonates at different frequencies under ferromagnetic resonance, showing that LSMO can be magnetically “tuned” without relying on conventional magnetic fields. This breakthrough marks a significant step toward neuromorphic circuits, as these materials can host diverse states in a single component, mimicking brain-like processing capabilities with far lower energy consumption.
The discovery complements existing work on voltage-tunable resistance in LSMO, suggesting that future spintronic neuromorphic devices could exploit both resistance and magnetism changes in the same material. By enabling robust yet efficient control over electron spins, scientists hope to build networks of “spin oscillators” for next-generation AI, sensing, and computing applications. This research was conducted by the Quantum Materials for Energy Efficient Neuromorphic Computing (Q-MEEN-C) Center, funded by the U.S. Department of Energy’s Office of Science, Basic Energy Sciences. Read more about it in DOE PAGES.
Patent update
Samsung and TSMC led USPTO granted patents in 2024
Samsung Electronics and TSMC have obtained the most granted USPTO patents of 2024, securing 6,377 and 3,989 grants respectively in 2024, according to data from IFI Claims. Samsung’s continued dominance, marking its third consecutive year at the top, represents a 3.44% increase from 2023, while TSMC’s second-place finish showcases 8.19% growth in patent grants. Asian companies now holding 56% of all U.S. patents. A recent article on R&D World also dug into the news, noting, for instance, that there is a growing concentration in electrical digital data processing CPC codes such as (G06F) and digital information transmission (H04L). Emerging technologies like battery technology (H01M) and organic electric solid-state devices (H10K) are also showing significant growth.